Collaborative Computing — How Web3 can be rocket fuel for enterprises’ data strategy
Artifact management
12 min readA Conversation with Jordan Brandt, CEO of Inpher
We are at the beginning of a fundamental reshaping of how data is used in the economy and Collaborative Computing is the next trillion dollar market. That is the premise of ‘The Age of Collaborative Computing’, a white paper written by Lawrence Lundy-Bryan, Research Partner at Lunar Ventures and a Nevermined collaborator, and who has conducted a series of interviews with 6 notable industry experts that present their expert views on the market evolution.
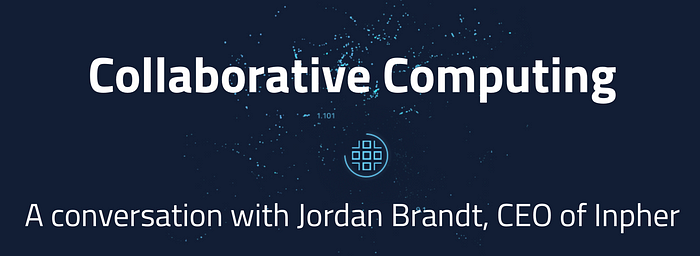
Currently, data is being recklessly underused. Barriers have rightly been put up by regulators around the world to limit the commercial exploitation of personal data. Now, with privacy-enhancing technologies (PETs) like federated learning, multi-party computation and homomorphic encryption, we are moving towards a state where we can create secure and multi-stakeholder ecosystems around data. When data can be safely shared, analyzed and built upon, the value of all data assets can be maximized for private and public value.
Why are we excited about this? Three reasons:
- Many of us at Nevermined have a background in Big Data and Analytics. We believe that Collaborative Computing will finally turn data assets into new value streams.
- We believe that this is a concept that will bridge the gap between Web3 ideas and Web2 realities.
- Plus, we believe that this is a killer use case for our Nevermined tech stack.
See, the core proposition of the Nevermined platform is that we’ve built a set of tools that enable developers to add utility to digital assets through the application asset interactivity.
When we say ‘digital assets’, a more encompassing term for NFTs, we don’t just mean ‘JPEGs on a blockchain’. In Web3 anything can be a digital asset. So we’re also talking about datasets.
And when we say ‘utility’, we don’t just mean ‘buy or transfer an asset’. We’re talking about injecting digital assets with advanced functionality, like dynamic payments, merging multiple NFTs to create remixes, or — check this out — setting up decentralized compute environments for in-situ federated learning on disparate assets.
It’s this concept of merging, slice’n dice, or remixing digital assets together that we believe will unlock their true latent potential. From this capability will arise more compelling collaborative opportunities, and result in stronger communal bonds. We capture this idea with what we call “Digital Asset Interactivity”.
As such, we believe Web3 technology and the idea of Asset Interactivity will play a major role in the ability to share and leverage data in novel ways and allow in the advancement of Collaborative Computing.
So to help us understand the challenge of establishing a new product category, we worked with Lawrence to create a series of interviews with experts in the data collaboration space who are buying, selling or investing in this vision.
Each of the conversations brings a different perspective and helps with understanding why Collaborative Computing is important to them and why we should care. These conversations reinforce our view that we’re at an inflection point. Transformations happen at the intersections of technologies and markets. At Nevermined, we excited to build the roads that lead there.
Happy reading.
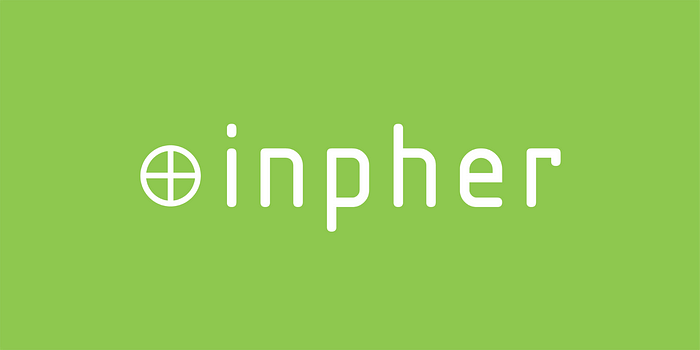
A conversation with Jordan Brandt, CEO of Inpher
By Lawrence Lundy-Bryan, Research Partner at Lunar Ventures
To explore the concept of Collaborative Computing in more depth, I spoke with Jordan Brandt, CEO of Inpher, a company pioneering privacy preserving machine learning. Highlights include:
- Why monolithic incumbents are not only missing performance and revenue opportunities, they are now going to be left with all the data risk;
- Why taking a vertical go-to-market strategy might be sub-optimal;
- Why data federation is an inevitability
When you pitch customers on making better use of their data, what benefits do you lead with?
As you say in the collaborative computing paper, we are selling solutions, not technology. So the reality is that the ROI depends on the specific problem the customer needs to solve. Different benefits persuade different customer stakeholders. The user is usually a data scientist and so, accessing more data is compelling, as is getting to work on data faster. Those benefits are of course important, but less so to the economic buyer, who obviously cares about cost and ease of integration and those sorts of things. Then you need sign-off from the CISO for security guarantees as well as legal who must make sure they remain compliant and understand all of the risks.
What is the most common barrier you encounter when selling your services?
The barriers are definitely falling. A few years ago the most common barrier was just market education. We spent a lot of time educating our customers and other decision-makers within the organization. That’s why we went with the term ‘secret computing’, because it’s easy to understand what you are getting. We made that market education piece easier. Then we spend a lot of time making it easy for users to persuade economic buyers within the organization. Everything from video explainers, case studies, factsheets, and all that stuff. Our job is getting easier, as we see more public work in the space. [[Editor: including what Nevermined is doing]]. But now we are finding the whole process of selling is easier and privacy-preserving tools are more common.
So now really the barriers are closer to typical SaaS selling. It’s all about how easy it is to spin up and use. How well does it connect with existing data pipeline tools? This is where privacy-enhancing tools still have the disadvantage because the fact is, it’s not as easy as a standard SaaS tool. We are using advanced cryptography and so we need to be much more careful about how it integrates with other systems. This process is much easier if our customer is already using a micro-services architecture, but it’s harder for organizations that are still using legacy systems. A banking executive said at a conference once that it’s “easier to build a new bank than to re-engineer the existing tech stack”. So I suppose this is a barrier for us, but more than that it’s a huge problem for companies using these legacy systems. In a few years they will be left not only with a slower, more expensive and less secure tech stack, but they won’t be able to protect their own and their user’s data either.
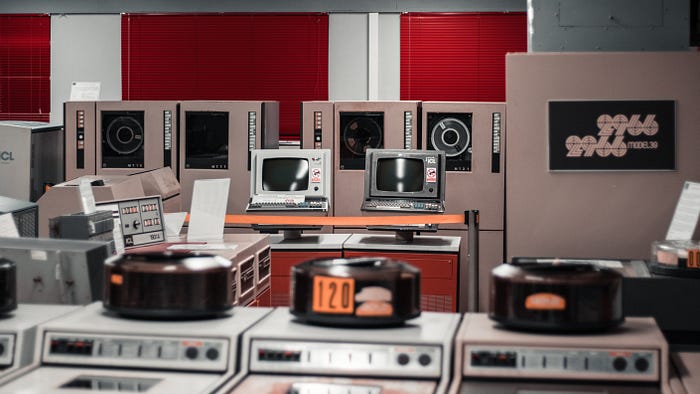
Enterprise data infrastructure sits across the entire company, who is the best person to sell to in your experience? Compliance seems like an easy way in but you could get stuck selling a commodity product competing on cost?
Yes that’s right, compliance was the place they started. They have a budget and a strong pain to solve with regulation. The next step was the CISO. They generally love our stuff because it reduces the risk they have to manage, we are shrinking the attack surface and limiting their data liabilities. So it’s powerful for them. But downside risk only gets you so far. Once companies start using Inpher, it becomes obvious that the people getting the most value are data scientists and, by extension, the commercial teams that are applying the algorithms for business value. So this is where we are now.
Which use cases do you think are the lowest hanging fruit for data collaboration tools?
I don’t know if it’s a case of finding use cases as such. Look, data collaboration or computing on encrypted data has widespread value. Every company wants to be more secure and leak less data. Every company wants access to more data or just uses all the data it already has. Really it’s a case of looking specifically at the jobs data scientists are doing today and making their lives easier. For us it’s about giving data scientists the tools to easily integrate into their workflows and then learning what algorithms and data types they want to work with. It’s more granular than market specific or even use case specific.
I’ve found financial services and healthcare to be relatively early adopters of data collaboration tools mainly because of the regulation around privacy and data security. Have you found the same and what other verticals do you expect to be the next adopters?
The obvious answer here is to say the best opportunities are in heavily regulated industries like financial services or healthcare. But that’s a little too crude. Financial services do have a greater technical understanding and budget to find ways to share data internally and externally. Healthcare, like financial services, has the need, but the sharing environment is just so complex. That said, pharma seems to be ahead of the game here. There is the obviously huge need and potential value unlock in sharing clinical trial data across the industry. And then the advertising industry has a relatively new but major need to find ways to target users in a private way. The advertising industry is not regulated to the same extent as financial services or healthcare, and so can experiment faster, which is a good thing for adoption.
All of this said, I don’t think vertical-by-vertical is the best way to uncover and target opportunities for data collaboration adoption. Just like use cases, the best way to think about this is from the data scientist perspective. What are there problems working with data today and what tools do they need to better do their job?
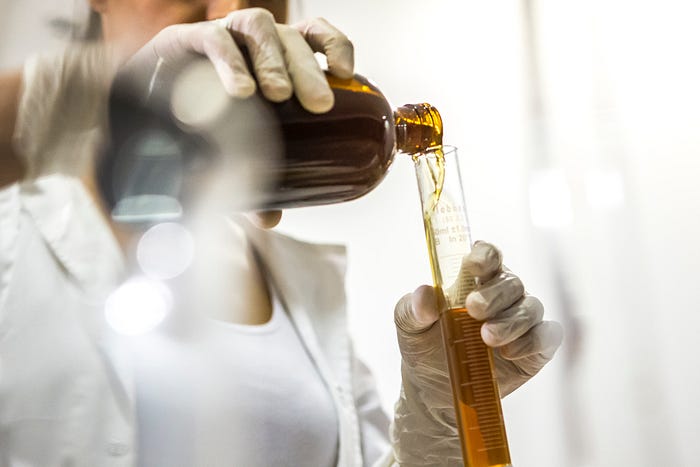
What cultural, technical or social change would be required for demand in data collaboration to increase 10/100x?
A 100x change can only be driven by consumer demand. I think the key is when users realize it’s a false trade-off between personalisation and privacy. It’s sort of framed today that you have to give up your privacy so you can get these personalized services. And people generally like personalized services and so the trade-off is worth it for most people.
But it’s no longer a trade-off we have to make. Secret computing and PETs generally mean that we can get personalisation without giving up our privacy. Now of course, we need some performance gains in the next few years so that plaintext and ciphertext processing is close to parity, but we will start to see that narrative. So people will ask: if I can get the same quality service without giving up my data then why not? You wonder at that point why all companies that use algorithms to deliver personalized services just won’t do private computing. There might be some small performance or cost trade-off, but the benefits in terms of PR, compliance, security and risk management will make it a no-brainer.
When thinking about helping companies utilize their data, a sensible framework is: governance, sharing, and monetization. It feels like 95% of companies investing in their data infrastructure are still on data governance, maybe 5% are finding ways to share internally, and <1% are even thinking about monetization yet. Does this sound right to you?
At a high level yes. Data monetization has been a promise since the advent of commercial PETs, technically we know this is where we need to end up to widely deploy this stuff. But the missing link has been the business model. And it’s not as simple as saying, let’s bundle up this dataset and set up some permissioning system to provide paid access. There are a whole bunch of complex questions around data control and ownership which aren’t simple. More pragmatically, very few companies are dedicating resources to systematically addressing these questions yet. We will know we are getting closer when we start to see new business units with general managers with teams set up to take data assets to market with a privacy-preserving layer. This is where forward-thinking CDOs are going, and we’re seeing this signal from companies who are already in the business of selling data.
We are seeing hundreds of startups looking to solve enterprise data problems, what do they need to know that you have learned the hard way?
Well I think your paper says it well: sell solutions not technologies. This is a particular challenge in cryptography because people who really understand the crypto obviously have to deeply care about the technology. Teams can understand that clients might not care as much as they do about the tech, but the primacy of the technology will come through in sales and marketing. So it is really important that culturally the organization empathizes with clients and helps them sell internally.
It feels like the data consolidation model that has been at the forefront of data utilization strategies has perhaps reached its limitations in terms of efficacy. With the emergence of “Data Mesh”, Collaborative Computing, and, more generally, customer centricity, do you see a horizon where a data federation model plays a more significant role in the lifecycle of data estates?
I haven’t personally come across the term data mesh yet, but as it relates to this trend towards decentralization and data federation, yes I see this. These architectural shifts are inevitable as new technologies emerge to enable new things. The reality is that the Cloud solved a whole bunch of challenges with distributed management of computing, storage and networking in a cost-effective way. Once it was all in one place it was much easier to analyze it and do statistics. We just didn’t have the tools to query or learn from distributed data until very recently. So we now have the tools, and the push is from regulation and national data sovereignty policies. Now it’s not inevitable to just bring everything together in one place to process. For many organizations, actually not centralizing data is a net benefit. So yes, a data federation model makes sense. But again, it’s important to note that the analyst doesn’t care about data federation. They care about availability, speed and time-to-deployment. So data federation will win in so much as it solves the everyday problems for customers.
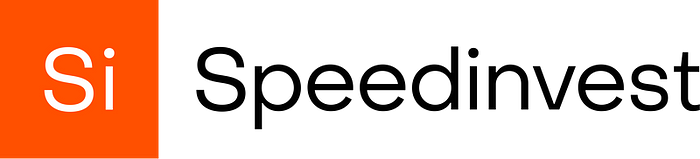
In the next part of this series, we’ll publish Lawrence’s conversation with Rick Hao, Partner at SpeedInvest. In that interview they’ll cover:
- Why getting more data will continue to be a business driver for the foreseeable future despite trends of cost-efficient algorithms and fewer data algorithms at the frontier;
- Why healthcare is likely to need a different data infrastructure than other markets and;
- Why machine learning will be the main driver of data sharing tools
And we’d love to hear from you. Got any questions, suggestions or want to chat about your project? Contact us via the website or join our Discord.
Originally posted on 2022-11-23 on Medium.