Partnership Announcement: Adding NVM Payments to FLock.io so AIs can pay, and get paid, for Federated Learning Models
AI models
5 min readFLock and Nevermined partner to make it easy to buy and sell FL and RAG based AI models.
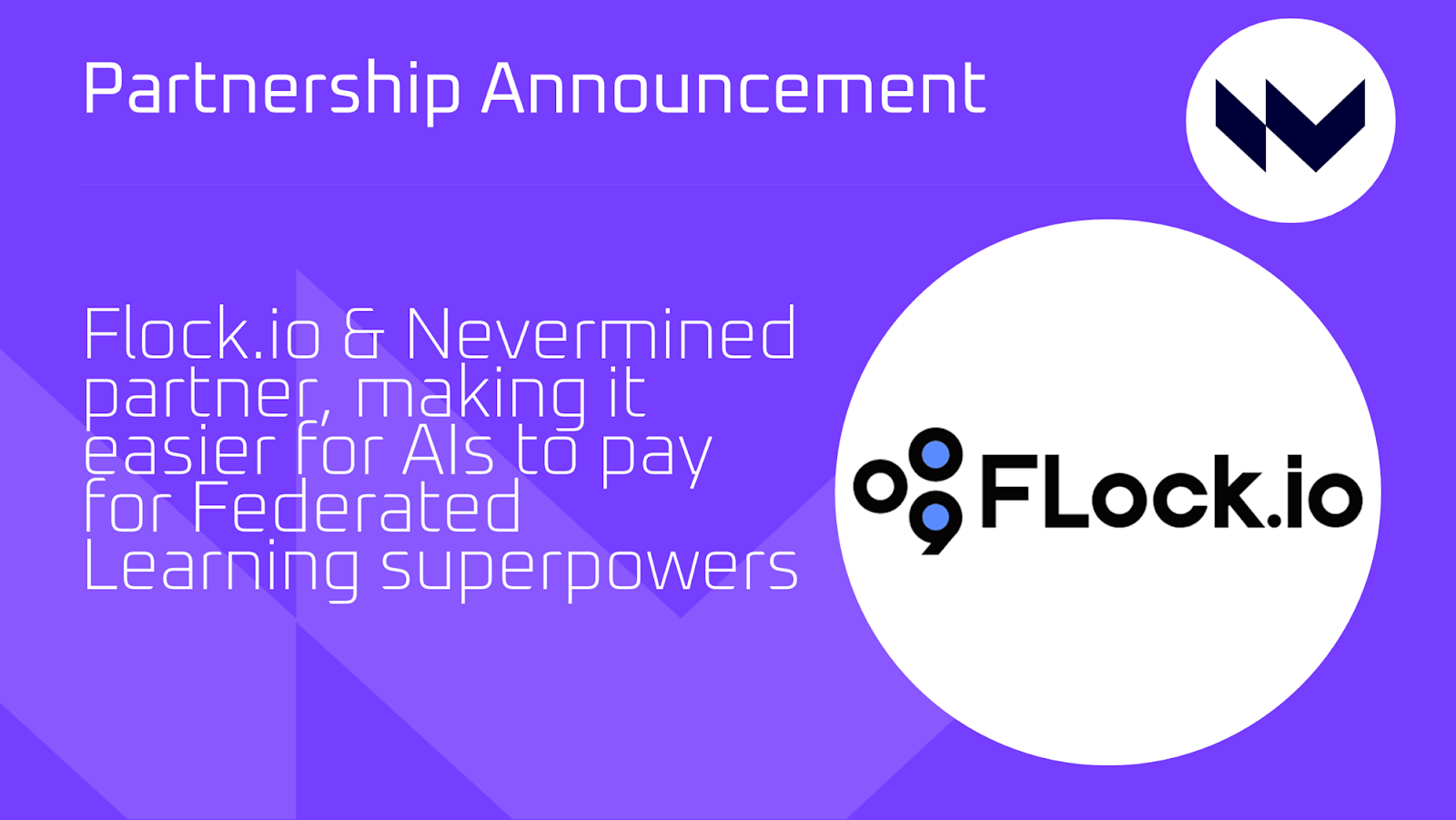
The ability to use datasets for AI modeling and Machine Learning, while keeping the data at rest, is an effort that is near and dear to the Nevermined team. Afterall, we pioneered the idea for Web3 at Ocean Protocol back in 2018. Back then we called it Compute-to-Data, now referred to by Filecoin as Compute-Over-Data, but which we call Data In-Situ Computation, mostly because it has the best acronym: “DISC”.
Regardless of the label, the idea remains the same: facilitate the deployment of models for algorithmic computation against datasets, all without moving the data. It’s a simple principle. Instead of moving the data to the compute (i.e. the contemporary way), you move the compute to the data (i.e. the federated way). This approach comes with massive benefits for collaborative computing and ownership, not to mention privacy.
Federated Learning…oh my.
Federated Learning is complex. It involves deploying models locally to run against distributed data repositories, all while ensuring responsiveness, completeness, and validity. Understanding where inputs are coming from, where outputs are going, and which models are being leveraged to produce these outputs is non-trivial at best. In the usual, centralized, command and control Machine Learning environment, authentication and authorization are tightly coupled attributes of the system. In other words, how each component or actor makes a request is highly managed and controlled.
In a Federated Learning environment, however, those same attributes need to be validated upon each request. Understanding who or what is making which request is critical to securing the validity of the model processing and its output. This requires incredibly complex track and trace systems to ensure valid operation.
Consequently, high fidelity provenance for tracking and traceability are a cornerstone of Federated Learning. And you won’t find a more elegant solution for establishing and maintaining provenance than a blockchain. Federated Learning buttressed by blockchain is a match made in heaven.
FLock – Federated Learning on the Blockchain
Enter FLock, the Federated Machine Learning Platform on Blockchain. By combining technologies, FLock is able to accelerate decentralized AI through on-chain governance that oversees collaborative training on local datasets. This means local datasets can be opened up to analysis by AI agents in a controlled manner. Leveraging the combined strengths of blockchain and artificial intelligence, FLock provides a powerful environment for model creation to organizations or individuals dealing with large, distributed datasets.
One of FLock’s goals is to enable AI co-creation, where the community owns finetuned and RAG based models that are developed through on-chain coordination. Together, the community will contribute data, algorithms, feedback and compute, and be rewarded in tokens for their contributions. This communal approach to finetuning and RAG development is revolutionary for AI systems.
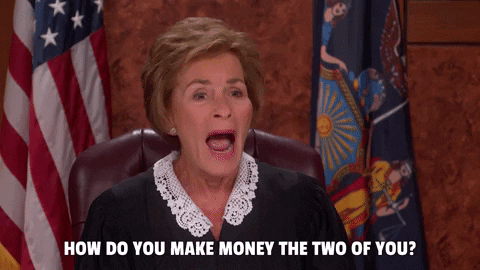
Rewarding and Monetizing AI Co-Creation
In addition to the complexity of building Federated / Decentralized Learning with on-chain coordination, there’s the issue of rewards and monetization. How do you reward multiple contributors of co-created models? Additionally, how do you handle monetizing a model with numerous non-homogeneous inputs, like data + algorithms + runtime environments? Identifying and evaluating what was provided by each contributor, implementing the reward and compensation logic, and then deploying the monetization mechanism is a task most centralized solutions handle poorly. And this doesn’t begin to touch on how an AI agent could access and integrate such models in a simple manner.
FLock + Nevermined Pay = Happy AI Models!
Building and maintaining a dynamic rewards and monetization system that handles the variable requirements of AI model co-creation and usage is complicated. It is also somewhat of a distraction from FLock’s core focus of creating a world-class decentralized model co-creation platform.
Solution: FLock partnering with Nevermined to provide these features to FLock builders, resulting in:
- better UX through dynamic rewards and payments and simplified AI agent usage;
- support for payment in a choice of crypto and / or fiat; and,
- an optimized approach that leverages the super powers of both teams.
With Nevermined Pay, FLock builders will be able to discreetly control access to their contributions, setting conditions like price, time period, and number of requests that can be made for their given contribution, whether it’s an AI agent, data, an algorithm, or an entire FL model. Builders can also work together to bundle their contributions via shared Smart Subscriptions, thus simplifying access to co-created models. Also, the integration will simplify AI agent consumption of FLock models by integrating a payment mechanism that all agents can use.
“Our partnership with Nevermined is a significant step towards achieving our mission of democratizing AI. By integrating their dynamic rewards and monetization system, FLock.io can now focus on creating a world-class decentralized model co-creation platform while providing our users with enhanced UX and streamlined access to co-created models. We are thrilled to join forces with Nevermined and look forward to the incredible innovations that will emerge from this collaboration.” – J Sun, Co-founder and CEO of FLock.io.
How Does it Work?
As an example, let’s say an AI builder wants to create a RAG based AI model that leverages their expertise in Credit Risk. The AI builder has a proprietary model that will run on a collection of credit histories, where this collection of credit histories is made up of individual credit datasets, each controlled by an independent Credit Risk modeler.
Using FLock’s platform, the AI builder can access each independent credit history dataset, and then deploy the builder’s proprietary credit risk algorithm to run locally on each dataset. The result is a highly accurate Credit Risk model that the builder wants to make available to others, for a price.
With the Nevermined integration, the FLock builder is able to set the price and access conditions to their model. For instance, the builder can set a price for access credits at $10 for 1000 credits, and then set the number of credits redeemed for their specific Credit Risk model to 100 credits per access request. When a user wants access to the model, they pay $10, and can subsequently access the builder’s Credit Risk model 10 times before needing to purchase more access credits.
What’s Next?
Over the next few weeks the FLock and Nevermined teams will work on integrating the Nevermined Pay solution into the FLock platform.
The motivation of both teams is to support AI builders in getting paid for their AI models in the simplest way possible without worrying about setup, integrating with payment APIs, credits accounting, user authorization, and so on. That way they can focus and do what’s best for them: creating amazing FL based AI models!
To find out more and stay up-to-date with everything Nevermined and FLock are working on, please join our respective Discords:
Nevermined: https://discord.gg/KPwZWqCS
FLock.io: https://discord.com/invite/ay8MnJCg2W